Boost User Experience with Personalized Video Recommendation Engine
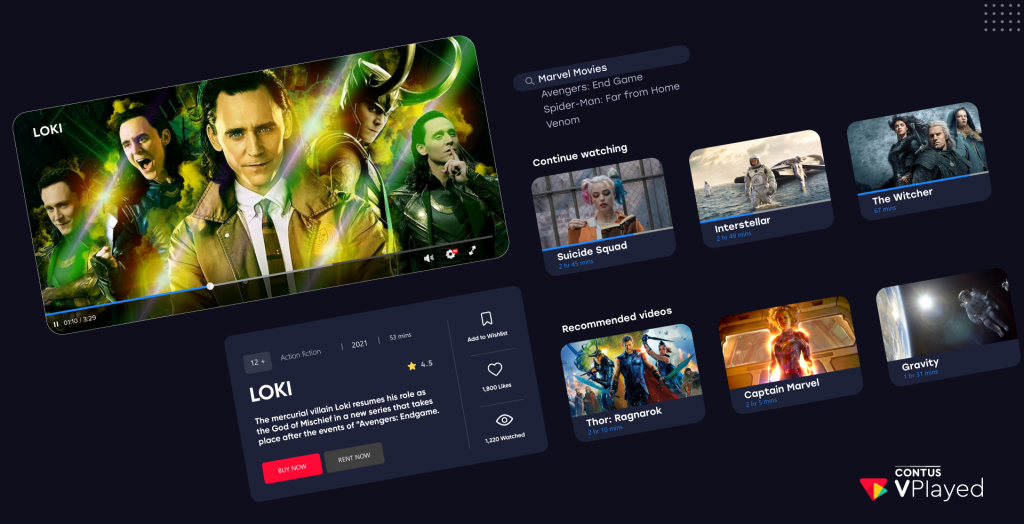
OTT is the big thing in the media industry. The way these platforms are leveraging the Internet to deliver content directly to the users is remarkable. One of the major reasons behind the success of new-age OTT Platform is that they allow the users to pay only for the type of content they would like to watch. They do not have to pay any extra bucks for irrelevant content that they might find monotonous and irrelevant. At the core of this system lies the concept of personalization.
Understanding user behavior is very crucial to succeed in today’s world of hyper-personalization. You need to understand the user behavior based on their browsing or viewing history and provide them with matching recommendations that they might like. Data science is at the core of analyzing user behavior in the digital world. You can analyze the user-behavior data gathered and make the most of your OTT endeavor with platforms offering smart recommendation systems with machine learning like that of CONTUS VPlayed’s.
One of the key reasons why personalization is important from a streaming business’s perspective is that it allows you to increase your revenue by segmenting your users and targeting them accordingly. In the contemporary era, the internet is widely accessible and affordable. This gives people a wide range of choices in terms of watching content on streaming platforms and also leads to information overload which can be overwhelming in most cases. Personalizing the streaming content for individuals will help you boost their satisfaction.
Table of Contents
Why Are Recommendation Engines Essential for OTT Platforms?
OTT platforms have personalized their offerings to suit the requirements of individual users. Personalization isn’t just limited to a subscription pack but it goes way deeper. Have you ever noticed Netflix or Prime Video suggesting a new set of movies/ TV shows just after you have watched a video? Well, these are personalized recommendations based on individual browsing history. Smart AI-powered recommendation engines even provide users with a percentage match to give a broad understanding of how that content matches their preferences.
Were you aware of the fact that almost 70% of the content watched on Netflix by users on the portal can be accredited to personalized recommendations offered by the OTT giant? The use of hyper-personalized recommendations is one of the key reasons for Netflix’s success in the streaming industry. These suggestions are nothing but highly calculated predictions based on facts and figures via user analytics.
Let’s delve deeper into two of the most prominent recommendation systems to understand how it helps.
-
Content-Based Recommendations
The content-based recommendation system takes into consideration the attributes of a content a user has consumed and recommends similar content that have the same attributes . Let’s take an example to understand this better. Suppose you’d searched for a movie in the past from a specific genre, market giants like Netflix will track it and offer you a whole list of best-rated movies in that particular genre. So, here they have made a prediction that you might like the best-rated ones in that segment and offered the same in the form of recommendations to watch.
-
Collaborative Filtering System
The collaborative filtering system works a little differently. This system recommends content based on how similar it is to your search history. You must have noticed ‘you might also like’ or ‘people also watched these’ sections on your favorite OTT platforms. These are examples of collaborative filtering systems into work. There are plenty of other recommendation systems used by streaming services to amplify the user experience and effectively market the content.
Advantages of Using A Recommendation Engine
A recommendation system can be explained as a tool to predict a user’s interest by leveraging data and turning the outcomes into options for the audience. The use of these engines helps companies to effectively market their products and services. It also helps to match consumers with best-suited offerings to boost their overall satisfaction. Let’s delve deeper to understand the most prominent benefits of using these systems.
-
Faster and Efficient Content Discovery
The first and most obvious benefit of using a recommendation system is that it helps users to discover content faster. Users can save a lot of time with effective recommendation systems in place to help them find relevant content quickly.
-
Title Recommendation on Home Page
As per a research report, it was found that only one-third of SVOD users explore the menu of a streaming website to watch content. As much as 21% of users reported that they quit watching if they are not able to make up their mind. Recommending interesting titles based upon the user’s preference on the home page allows users to easily decide what to watch.
-
Binge Streaming Functionality
Binge streaming or binge-watching can be explained as a process of watching any form of content for a prolonged period. Have you ever continuously watched your favorite show on Netflix without worrying about anything else? Well, that’s an example of binge-watching. Smart recommendation systems help to boost binge streaming functionality by providing the most suitable content that users find hard to skip.
-
Time-Based Suggestions
Most of the users usually binge watch on the weekends when they are free from work. A smart recommendation system can help to provide time-based suggestions and promote binge-worthy content on the weekends. This also helps to maintain subscribers’ loyalty as they don’t have to bother themselves with finding binge-worthy content to watch.
-
Valuable Advertising
Dynamic recommendation engines also boost the marketing ROI for advertisers. It helps them effectively target the right audience on the right platform. They show ads to users based on their browsing history and content preference. While a content-based filtering system relies on leveraging the characteristics of the product to make recommendations, a knowledge-based recommendation system uses function knowledge to draw a connection between a user’s need and a suitable product.
-
Real-Time Data to Decrease Churn Ratio
Data is the real deal in the modern era. Dynamic recommendation systems can effectively utilize user data including their search or browsing history to decrease the churn ratio. Churn rate is explained as the percentage of subscribers (usually for a service) who discontinue a subscription within a particular period. Efficient content discovery systems help to provide a personalized experience to the users and reduce the churn rate to a great extent by increasing customer satisfaction.
-
Subscriber Retention
Would users subscribe to an OTT channel if they don’t find suitable content to watch even after searching for hours? Audiences won’t see it as a good investment and will most likely start looking for alternatives. With the use of a smart recommendation engine, streaming services can suggest the most suitable content for a user to watch which in turn reduces churn and helps in user retention.
In the present age, users have a plethora of options to choose from but are restricted by personal time constraints. They want to make the most of their limited leisure time. Some of the prominent advantages of using a smart recommendation system including efficient content discovery, time-based suggestions, title suggestions on the home screen, etc will help you improve the user’s experience and boost their satisfaction. You can easily achieve this using the revolutionary solution, CONTUS VPlayed that will help you improve the user experience quotient.